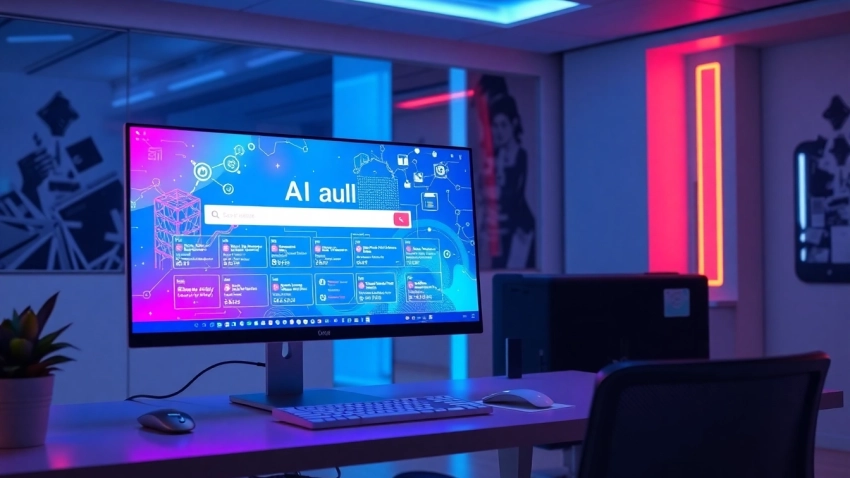
Unlocking the Power of AI Search: Transform Your Online Experience
The Evolution of AI Search
History of AI in Search Technology
The evolution of AI search technology dates back to the early days of the internet when search engines like AltaVista and Ask Jeeves aimed to organize web content. However, as the amount of available data exploded, so too did the complexity of the search process. The introduction of machine learning algorithms brought a transformative change, enabling search engines to analyze data at unprecedented speeds and accuracy. Innovations like Google’s PageRank algorithm changed the landscape, allowing for an understanding of relevance and ranking that went beyond mere keyword matching, laying the groundwork for future advancements.
As machine learning progressed, the integration of AI became more pronounced. The advent of natural language processing (NLP) allowed search engines to interpret queries more intelligently. This technology helps understand user intent rather than relying strictly on keyword matches, significantly improving the user experience. As we moved into the 2010s, AI capabilities expanded rapidly with major companies like Google and Microsoft investing heavily in what they termed “smart search,” characterized by personalized results and context-aware recommendations.
Current Trends in AI Search Engines
Today, AI search engines are embracing multimodal functionalities. This means they can process and integrate various data types such as text, images, and voice, allowing for a richer search experience. Google’s Gemini and Microsoft’s Copilot are prime examples, effectively streamlining the user experience by offering solutions that cater to specific queries without requiring a predefined path through a linear set of search results.
Another critical trend is the rise of personalized search experiences. Leveraging user data, AI search engines can tailor results based on previous interactions or preferences, leading to a more engaging and efficient search process. For instance, AI models can analyze user behavior and modify search outcomes accordingly, enhancing relevance and satisfaction.
Future Predictions for AI Search
Looking ahead, it is anticipated that AI search engines will become even more sophisticated with continuous advancements in NLP and machine learning technologies. One potential development includes enhanced contextual understanding, where AI could predict user needs based not only on their current query but also on historical interactions and trends. The integration of AI with augmented reality (AR) and virtual reality (VR) could also revolutionize how we access information, with immersive search experiences becoming commonplace.
Furthermore, as AI ethical considerations come to the forefront, we can expect developments in transparency regarding how AI models function, especially how they source, analyze, and rank content. These advancements aim to foster trust and reliability in AI-driven outcomes, which is essential as reliance on AI grows across industries.
Understanding AI Search
What is AI Search?
AI search refers to search technologies that employ artificial intelligence techniques, such as machine learning, natural language processing, and predictive analytics, to provide more relevant and contextual search results compared to traditional search engines. The goal is to enhance the overall search experience by understanding the nuances in language, user intent, and real-time feedback.
How AI Search Works
AI search engines utilize various methodologies to process and analyze data. At the core, these engines collect massive amounts of data to inform their machine learning models. NLP techniques enable them to extract meaning from user queries and content, allowing them to understand context, sentiment, and intent.
Once a query is entered, AI models leverage algorithms that assess various factors, including relevance, popularity, and freshness of content. They also evaluate user data to personalize results, ensuring that users receive the most relevant information based on their distinct search histories and preferences. This results in search experiences that feel more intuitive and tailored to individual needs.
Types of AI Search Engines
AI search engines can be categorized into several types based on their capabilities:
- Text-Based AI Search Engines: These engines focus on parsing text data to provide relevant search results, employing sophisticated algorithms to rank information based on language understanding.
- Visual Search Engines: Utilizing image recognition technology, these engines allow users to perform searches based on visual data, facilitating searches through pictures rather than keywords.
- Voice-Activated Search Engines: These AI search engines respond to spoken queries, making information retrieval more accessible through devices like smartphones and smart home assistants.
- Context-Aware Search Systems: Leveraging user data and behavioral analytics, these applications can adjust the search results based on user location, preferences, and time of day.
Benefits of Using AI Search
Enhancing User Experience
The primary advantage of AI search is its ability to significantly enhance user experience. By employing natural language processing, AI search systems can deliver results that resonate with the user’s intent rather than just the textual match of keywords. This leads to a more engaging and efficient search process, where users find relevant information quicker and with less effort.
Additionally, AI search tools adapt over time. By learning from user interactions, they continue to improve, which means that the more you use them, the better they get at understanding your preferences and needs. This ongoing enhancement leads to a dynamic and user-centric experience that evolves with the user.
Speed and Efficiency Gains
AI search technologies dramatically improve the speed and efficiency of information retrieval. Traditional search engines often require users to sift through multiple pages of search results, while AI-driven solutions bring relevant answers right to the forefront. With advanced algorithms and indexing techniques, users can receive meaningful information almost instantaneously.
This is particularly beneficial for businesses where time is of the essence. Employees can access a repository of information quickly, reducing downtime and allowing them to focus on critical tasks. AI search engines can parse vast datasets and return results that are immediately actionable, which can lead to enhanced productivity across the board.
Personalization through AI
One of the most significant advantages of AI search engines is their ability to deliver personalized content. By analyzing user data, behaviors, and preferences, these engines can craft search experiences that resonate on an individual level. Whether it’s presenting results based on past searches, geographical location, or predicted interests, AI search creates a tailored environment that enhances the likelihood of user engagement and satisfaction.
This personalization can lead to better decision-making, as users receive information that is directly applicable to their queries rather than generalized content that may not serve their needs. Businesses can leverage this personalization to improve customer service, enhance product offerings, and increase overall consumer satisfaction.
Challenges in AI Search
Data Privacy Concerns
As the reliance on AI search engines grows, so too do concerns surrounding data privacy. These systems often require access to extensive user data to function effectively, which raises questions about how such data is collected, stored, and used. Users may feel uncomfortable sharing personal information, and organizations must ensure compliance with regulations like GDPR and CCPA.
Finding a balance is crucial; companies must adopt transparent data policies that inform users about what is being collected and how it benefits them. The development of ethical guidelines around AI usage will not only build trust but also enhance the long-term viability of AI search technologies.
The Issue of Bias in AI Models
Another significant challenge within AI search is the presence of biases in the underlying AI models. Because these technologies learn from historical data, they can inadvertently perpetuate existing biases present in that data, leading to skewed search results. For instance, certain demographics may receive less representation, resulting in a lack of relevant results for those users.
Addressing bias in AI requires ongoing assessment and improvement of training data, ensuring diverse and representative datasets. Organizations must also put measures in place to regularly audit algorithms and adjust them as necessary to promote fairness and equity in search outcomes.
Limitations of Current Technologies
Despite their advancements, AI search technologies still face limitations. For example, language variations, slang, and idiomatic expressions can pose challenges in natural language understanding. While AI has made significant strides, encore examples still exist where it misinterprets user intent, leading to irrelevant results.
Moreover, knowledge representation remains tricker for machines. Complicated queries may not be entirely understood, resulting in missed information or an incomplete understanding of the topic at hand. As the technology evolves, continuous enhancements in NLP and contextual understanding will be essential to rectify these limitations.
Implementing AI Search in Your Business
Choosing the Right AI Search Solution
When selecting an AI search solution for your business, it is vital to evaluate options based on your unique needs. Considerations should include scalability, integration capabilities with existing systems, and support for multiple data types. Engage in a thorough assessment of the available technologies, such as Azure AI Search for enterprise-oriented solutions or specialized tools for specific industry needs.
Moreover, prioritize platforms that emphasize user-friendliness, enabling employees without extensive technical knowledge to maximize the software’s capabilities. Customer reviews and case studies provide valuable insights into the effectiveness of various AI search engines, aiding in informed decision-making.
Integrating AI Search with Existing Systems
Integrating AI search solutions into existing systems can be a complex yet rewarding endeavor. It is essential to conduct a thorough analysis of data architecture to ensure compatibility and seamless operation. Create a gradual implementation plan that allows for testing and adjustments, reducing potential disruptions to business processes.
Collaboration between IT and business units will be crucial for successful integration. Collecting feedback from end-users during the testing phase can help identify any gaps or issues early in the process, allowing organizations to make necessary adjustments before full deployment.
Measuring Success and ROI in AI Search
To gauge the success of AI search implementations, businesses must establish clear metrics and key performance indicators (KPIs). Metrics such as reduced search time, user engagement levels, and satisfaction ratings can offer insights into the technology’s value.
Additionally, it’s essential to monitor the financial impact of implementing AI search. Analyzing cost reductions associated with operational efficiencies, increased sales through enhanced customer engagement, and overall productivity improvements can help showcase the return on investment from AI search technologies. Regular audits of search performance should be conducted to ensure the system continues to meet business objectives and user needs.
Leave a Reply